Environmental variation, which occurs in most habitats, must be taken into account in the experimental design. Under field conditions, it is usually impossible to exert control over the large numbers of independent variables which introduce heterogeneity into the experiment. Such heterogeneity is usually important because researchers are interested in testing the predictive power of the hypotheses on the overall weed population, not just a homogeneous subset of individuals.
Spatial arrangement of the experiment is important. It is not valid to have some of the treatments at one site and the rest somewhere else. Clearly, if treatments are carried out at different sites then it is impossible to know whether any differences are due to the treatments themselves or to the differences between sites.
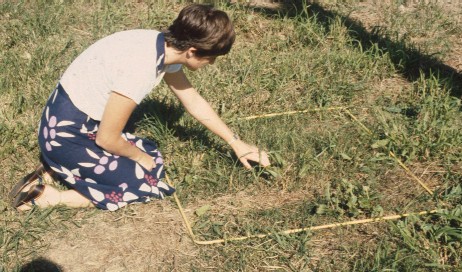
A biological control field experiment using quadrats to
collect
data.
|
All treatments should be applied across the same range of heterogeneity. Successful experimentation involves accounting for as much variation as possible, leaving the minimum unexplained. Variation exists in every system, even before we apply treatments, particularly in field experiments. Experimental blocking is a very efficient way of accounting for many kinds of spatial, temporal and operator variation. For example, if you suspect that there is an environmental gradient across the site, (say in slope, hydrology, nutrients, or shade), then blocks should be laid out along the supposed gradient. Even if spatial differences are not obvious, they always exist and it is therefore still useful to set up the experiment in blocks. The blocks should all be large enough to contain at least one repetition of every treatment. Blocks should be as compact (i.e. square) as possible, but if rectangular, the long axis should be at right angles to the gradient to minimise the within-block variation. The plots for the different treatments should be placed as far as possible side by side, along an axis at right angles to the gradient. Treatments can then be allocated at random to plots within each block. Such an experiment, called a randomised block design, can then be analysed by analysis of variance. The effects of environmental heterogeneity can be removed from the overall variation as the block factor when carrying out the analysis
(See Snedecor and Cochran 1980).
Blocks can be used to allow for temporal and operator associated variation as well as spatial variation. If all replicates cannot be applied at the same time, complete blocks should be set up together to avoid variation due to time between treatments. If each operator works in the same block on each sample occasion, systematic variation between operators in the way they measure or apply the treatment will be added to differences between blocks rather than differences between treatments.
Within a site, recognition of micro-habitats is important. For example, plants standing in free water will have different properties from those not submerged. Also, isolated plants have a very different growth habit from plants within a stand. By using blocking, or by pairing plants within a micro-habitat, heterogeneity between treatments is reduced and the experiment can include a representative array of plants on which to test the hypothesis.
If environmental variability is high, the number of replicates (i.e. blocks in a randomised block design) should be increased so that treatment effects will still be detectable against the heterogeneity. It is possible, and very good practice, to determine the minimum number of replicates required for an effective experiment before setting it up. This requires:
 | some idea of the variation inherent in the material (the coefficient of variation or CV) and, |
 | an idea of how big a difference between means one is interested in
detecting. |
We can then use tables (available in
Cochran and Cox
1957), or statistical software (e.g. Sigmastat from Jandel Scientific
- now part of SPSS) that will estimate how many replicates are required to obtain a statistically significant result. As the CV increases, the required number of replicates increases. Similarly, if we need to detect very small differences between treatments, this will also require more replicates. The CV could be estimated from preliminary studies on the organism in question, or from the literature, or from previous experience with similar organisms. The size of the difference we need to detect can be a subjective decision, but is better if arrived at objectively. For example, if there is information on the economic threshold of the weed (i.e. to what density it must be depressed in order for satisfactory control to be achieved), we could use this to establish the size of the difference to be detected. Clearly, to estimate the number of replicates required, we may have to make some assumptions. Provided these assumptions are clearly stated, however, this is far better than simply a stab in the dark that might result in too few replicates or too many being set up. In either case resources are wasted.
Covariance analysis is another technique used to reduce experimental error and increase sensitivity in designed experiments. Essentially, one measures the background variation for the variable in question in order to remove it from the analysis so that one is left only with the difference due to the treatment. For example, individual shrubs vary widely in seed production. If we are to apply an insecticide treatment to twenty shrubs that produce seeds annually, with twenty as controls, a covariance design would involve measuring the seed production for all forty shrubs in the year before the application of the treatment. One then uses the previous
year's seed production as a covariate in the analysis. If we do not have the resources to measure this in the year before, we can at least measure the size of the shrub in the year of treatment, because this will explain much of the variation in seed output, irrespective of whether the plant is a control or treatment plant.
There are situations where replication is simply not possible. Perhaps we can only afford one treatment lake to receive biological control agents and one control lake for comparison. In this situation, Hurlbert
(1984) recommends that we avoid inferential statistics like ANOVA,
t-tests and X2 (chi-square) tests and instead simply present the means and the variation around the means for the two systems. Inferential statistics are not applicable and would not make the results any clearer. One can merely draw qualified conclusions about the treatment in question, but there is no true replication so no
P value can be calculated.
[ Back ] [ Next ]
Grant Farrell and
Mark Lonsdale
|
|